Here’s what early-adopters have to say about ACES!
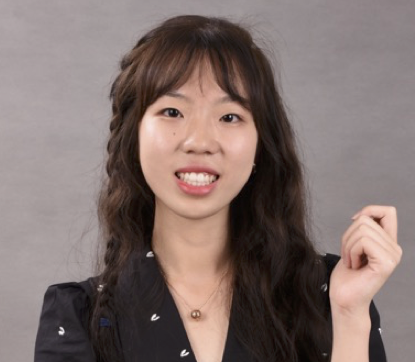
Ruisi Cai (UT-Austin) uses ACES to process long context sequences in Large Language Models (LLMs). “Due to transformers’ quadratic memory requirements, LLMs command substantial computational power and agile memory management,” said Cai. The UT-Austin team developed a unique approach that was highlighted in a paper titled, “Learning to Compress Long Contexts by Dropping-In Convolutions.” Their paper was accepted by the International Conference on Machine Learning (ICML24).
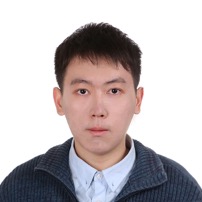
Aocheng Li (Purdue) uses ACES for data-driven archaeological site reconstruction. They said, “I love its elegant and light-weight web interface for file manipulation and job creation/submission. Using the composability features, I combine virtual network computing and TensorBoard servers to launch jobs and monitor training output with just a few clicks - all within one browser session. The HPRC staff are extremely helpful, and are quick to solve my issues and concerns. Using ACES has been an enjoyable experience.”

Freddie Witherden (Texas A&M Department of Ocean Engineering) used ACES to perform high-order accurate fluid flow calculations of bluff bodies. “The range of hardware, including CPUs, NVIDIA GPUs, and Intel GPUs, is perfect for the development, testing, and evaluation of performance-portable coding paradigms. Additionally, the large-memory nodes have proved invaluable for enabling us to perform preprocessing work for simulations on leadership-class computing resources.”
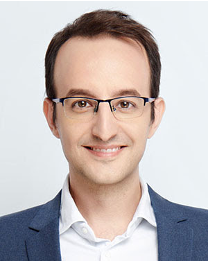
Rubem Mondaini (University of Houston) uses ACES to study quantum many-body problems in Condensed Matter Physics with the goal of understanding how Coulomb repulsion between electrons can affect quantum matter topology. "ACES’ abundant supply of the latest CPUs (Sapphire Rapids), large memory and fast interconnect make it possible to reach physical system sizes unforeseen until now," said Dr. Mondaini. “This unique combination of assets makes all the difference with investigations in the quantum world,” he added.
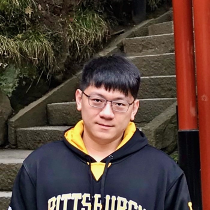
Chen-Chun Chen (Ohio State University NOWLAB) primarily uses the Intel GPUs and XeLink nodes on ACES. “Using TensorFlow and Horovod, I’ve been running OSU Micro Benchmarks (OMB) to extend the MVAPICH library to support Intel PVC GPUs,” he said, and added, “I receive invaluable assistance from the HPRC helpdesk, and my experiments on ACES have been consistently smooth.”
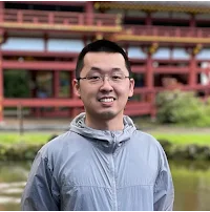
Junyuan Hong (UT-Austin) cited ACES in his latest research which presents a new method for private prompt tuning of LLMs, like ChatGPT. The solution is called Differentially-Private Offsite Prompt Tuning (DP-OPT) which employs a discrete client-side prompt that can be applied to desired cloud models without significantly
compromising performance.
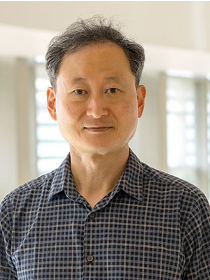
Wonmuk Hwang (Texas A&M Department of Biomedical Engineering) performs molecular dynamics simulations of biomolecules - a task best performed with state-of-the-art computational resources. Dr. Hwang uses ACES to investigate the mechanical response of T-cell receptors which defend against pathogens like influenza and the SARS CoV-2 virus that was responsible for the COVID pandemic. “The NVIDIA H100s are great for carrying out multiple simulations, and the HPRC staff are always helpful when troubleshooting aspects of this novel testbed,” he said.
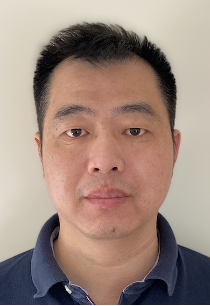
Hanning Chen (Texas Advanced Computing Center) used ACES to conduct a Molecular Dynamics (MD) simulation of Satellite Tobacco Mosaic Virus with more than 28 million atoms. “MD simulations of large biological systems are significant because they reveal functions contributed by millions of atoms, or more,” he said, and added, “Our benchmark test with NAMD3 and a 64-node run revealed a performance of 4.8 ns/day, with an impressive 80 percent scaling factor when we increased the number of nodes from 1 to 64. ACES is a powerful tool for MD simulations, and the HPRC support team’s knowledge of this novel platform helps researchers progress quicker.”